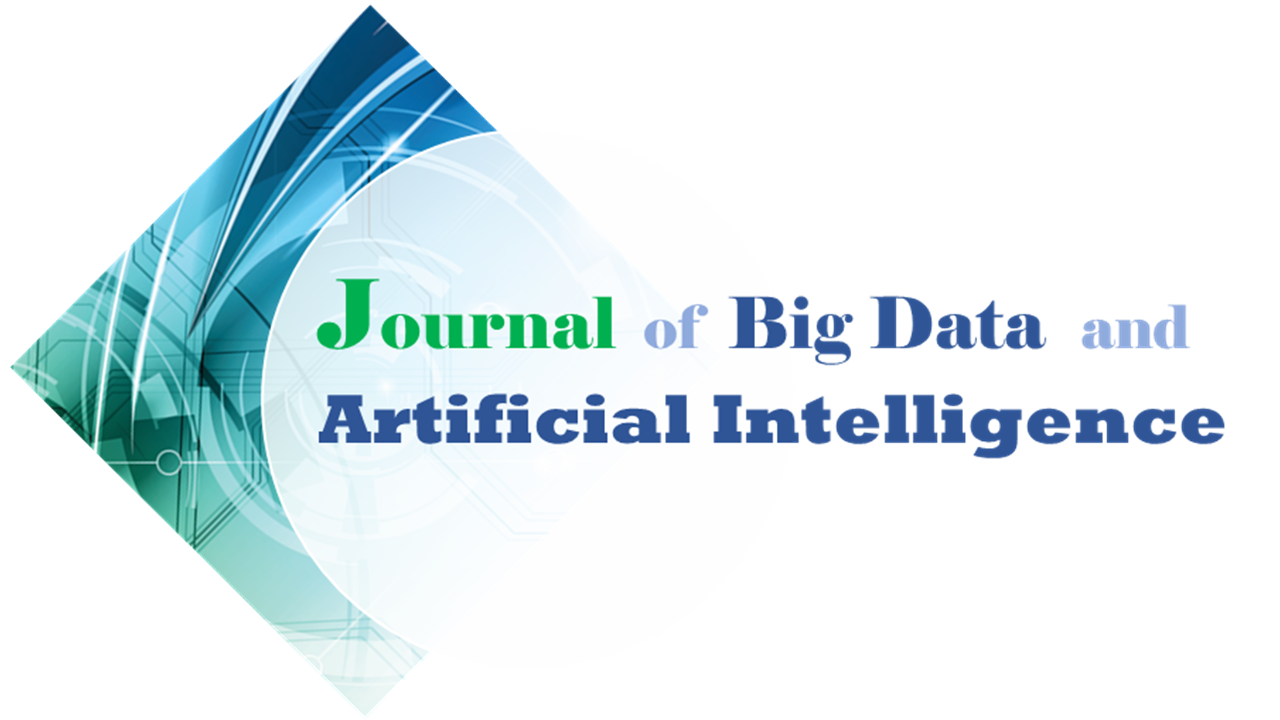
This study works on the development of a Generative AI University Support System (GenAI-USS) by improvising retrieval augmented generation (RAG) architecture to improve the performance of Large Language Models (LLMs) in a way that supports step-wise transparency. We aim to achieve improved accuracy of responses to queries based on university data assimilated from university webpages and knowledge sources. We employ RAG to develop a plug-and-play mechanism along with prompt selection to boost LLM accuracy. One of the key components in our GenAI-USS is the capture and integration of real-time information via live retrieval into the generative AI process. This domain-specific knowledge assimilation with real-time updates to capture changes and new information serves as a specialized dynamic expert knowledge database for RAG. Our RAG mechanism pulls in relevant, up-to-date information from the dynamic database, which pulls real-time data from targeted predetermined knowledge sources. The other key component in our GenAI-USS design is the deliberately designed information processing visibility at each stage of the process to ensure full transparency, and this includes: overview, data collection, storage encoding, testing, chatbot interaction, and search. The testing module allows for interactive viewing of generated responses and their sources. Our strategy is expected to lead to higher-quality AI-generated output via targeted information retrieval, hallucination mitigation, accuracy improvement, and timely data updates. Essentially, on the submission of a query, the RAG-dependent GenAI-USS first identifies the most relevant information from the specialized expert knowledge database and then factors this into the generative AI response development process. This results in a transparent RAG-based generative AI process with notable improvements in the quality of output produced.