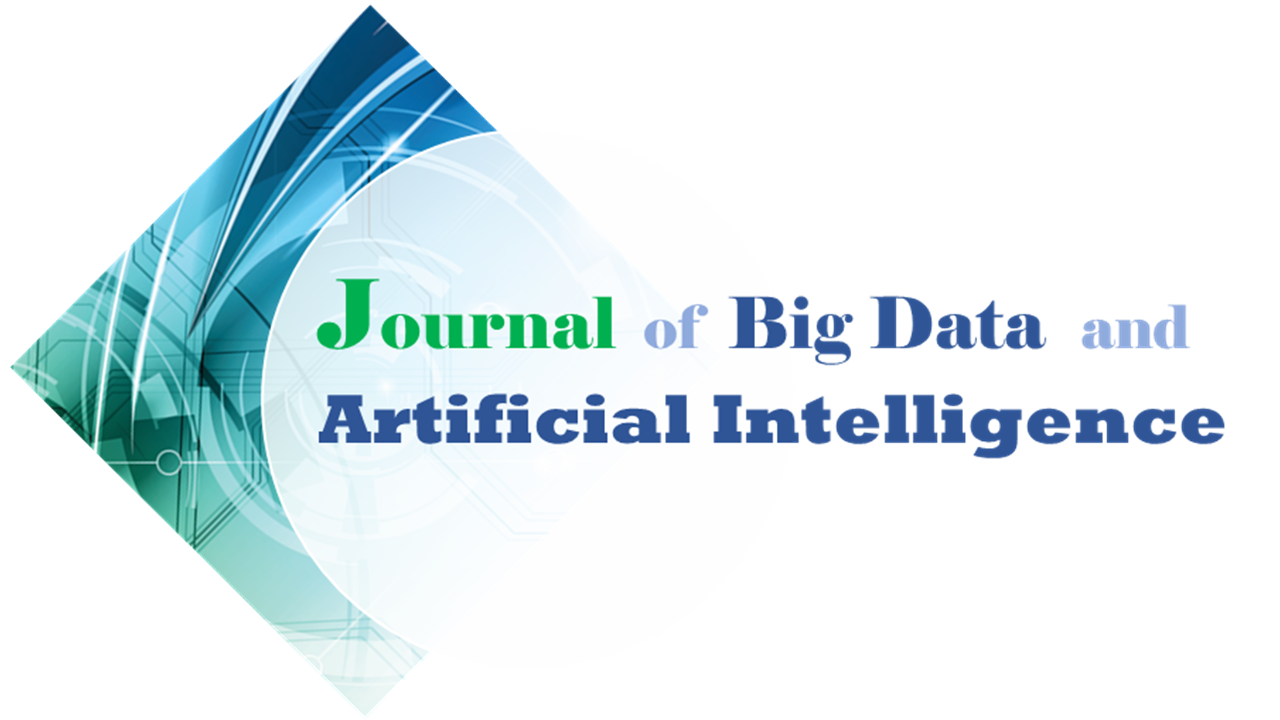
Predictive analytics has immense potential in disease type classifications. The key is to identify the set of genetic and clinical variables that can serve as predictors for disease classification purposes. However, the predictive and the prescriptive models both suffer from high dimensionality of these predictors. Therefore, it becomes important to identify a subset of these genetic and clinical variables that can be used for disease type predictions. Earlier studies have identified a subset of 978 landmark genes that can infer the expression values of the remaining gene in the human genome with ~81% accuracy. This study focuses on understanding if there is any significant difference in the characteristics of the landmark and non-landmark genes. Several experiments were performed on diseased tissues that were classified across race, ethnicity, and disease types with an objective to identify the number of differentially expressed genes within the landmark and non-landmark gene sets. Statistically, there was no conclusive evidence to support the hypothesis that there is a significant difference in the number of differentially expressed genes across the landmark and non-landmark gene sets.