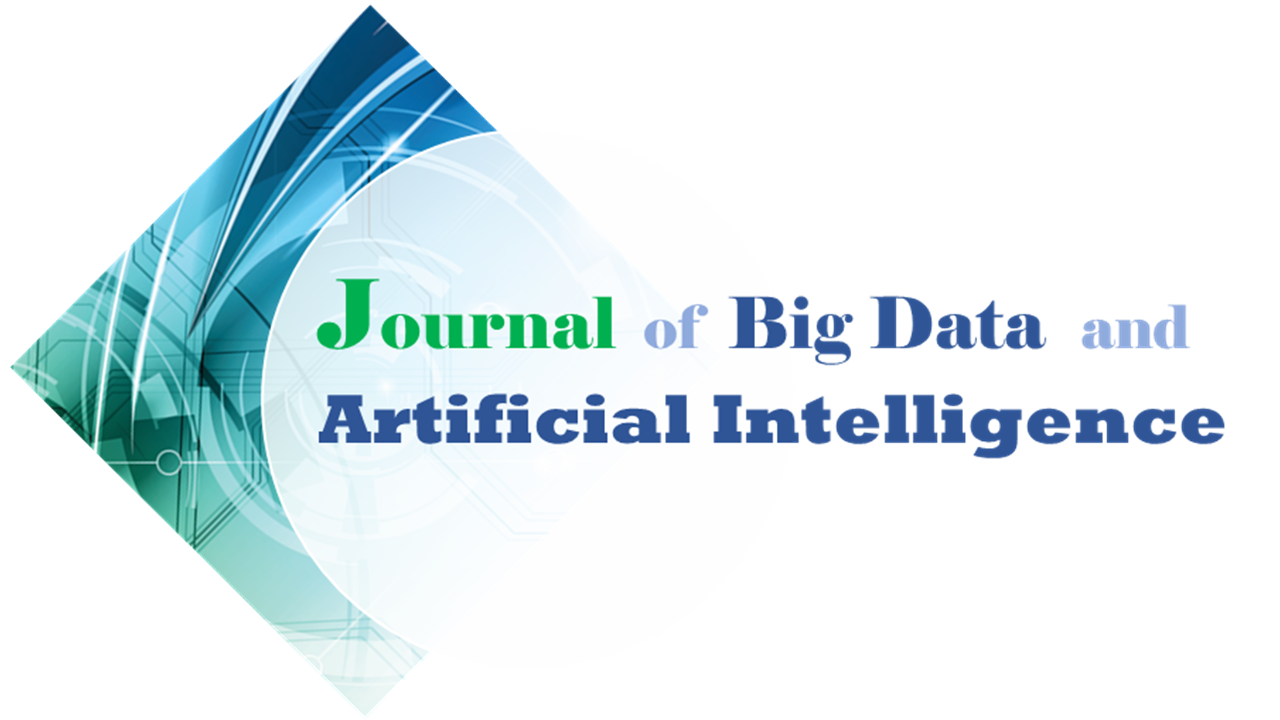
This paper presents a new approach for detecting fake news on social media. Previous works in this domain have demonstrated that context is an important factor when attempting to distinguish subtle differences within text. Fake news itself presents different level of difficulty due the vast similarity that exists between genuine and fake news contents. Therefore, we propose a collaborative approach which uses probabilistic fusion strategy to combine the knowledge gained from modelling two language models, BERT-LSTM and BERT-CNN. To achieve the fusion, we exploit the Bayesian method. Our experiments are conducted on two fake news detection datasets. The detection accuracy attained in these experiments attest to the efficiency of the proposed method, as our approach is very competitive compared to the state-of-the-art methods.